Visible to Intel only — GUID: GUID-51614DEE-9DF8-4D45-80F5-3B25CB7FF748
Visible to Intel only — GUID: GUID-51614DEE-9DF8-4D45-80F5-3B25CB7FF748
Error Analysis
In practice, most computations are performed with rounding errors. Besides, you often need to solve a system Ax = b, where the data (the elements of A and b) are not known exactly. Therefore, it is important to understand how the data errors and rounding errors can affect the solution x.
Data perturbations. If x is the exact solution of Ax = b, and x + δx is the exact solution of a perturbed problem (A + δA)(x + δx) = (b + δb), then this estimate, given up to linear terms of perturbations, holds:
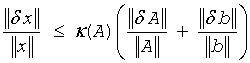
where A + δA is nonsingular and
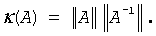
In other words, relative errors in A or b may be amplified in the solution vector x by a factor κ(A) = ||A|| ||A-1|| called the condition number of A.
Rounding errors have the same effect as relative perturbations c(n)ε in the original data. Here ε is the machine precision, defined as the smallest positive number x such that 1 + x > 1; and c(n) is a modest function of the matrix order n. The corresponding solution error is
||δx||/||x||≤c(n)κ(A)ε. (The value of c(n) is seldom greater than 10n.)
Machine precision depends on the data type used.
Thus, if your matrix A is ill-conditioned (that is, its condition number κ(A) is very large), then the error in the solution x can also be large; you might even encounter a complete loss of precision. LAPACK provides routines that allow you to estimate κ(A) (see Routines for Estimating the Condition Number) and also give you a more precise estimate for the actual solution error (see Refining the Solution and Estimating Its Error).