Intel Newsroom Archive 2020
December 31, 2020
Published
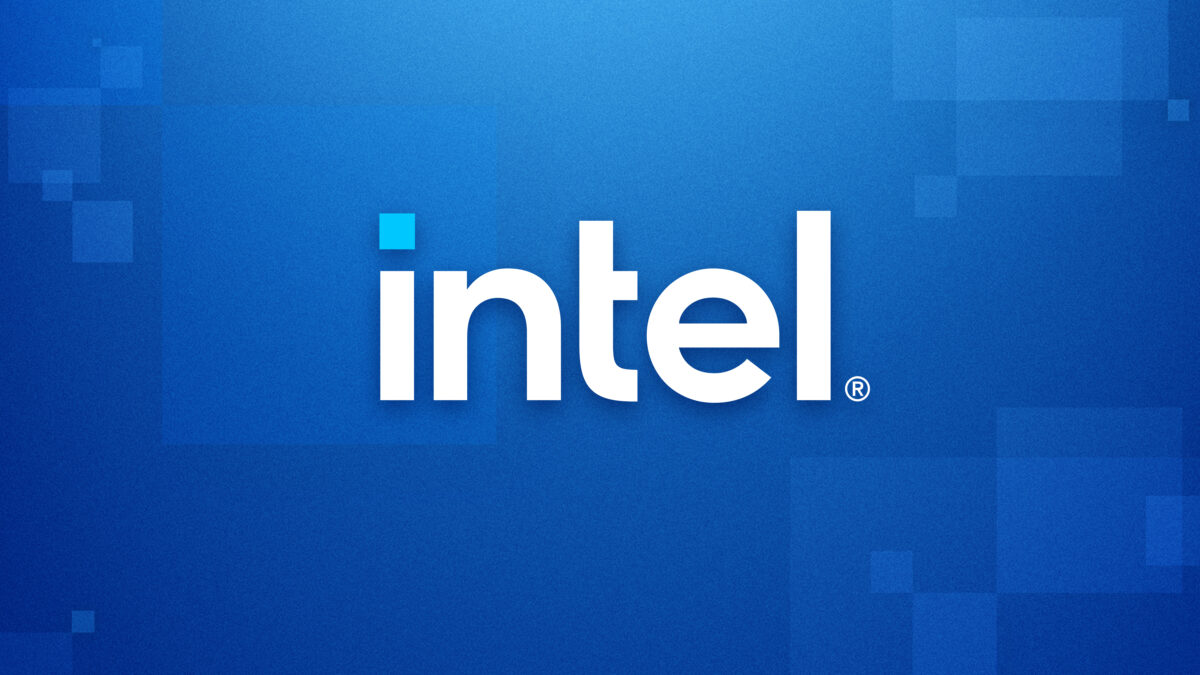
List of Intel Newsroom articles and news from 2020
In this article:
-
- January 2, 2020: In the News Engadget Offers Inside Look at Intel's EUV Technology
- January 6, 2020: 2020 CES Intel Brings Innovation to Life with Intelligent Tech Spanning the Cloud, Network, Edge and PC
- January 6, 2020: 2020 CES Intel News Conference - 'Innovation through Intelligence' (Replay)
- January 6, 2020: 2020 CES The American Red Cross and Intel Use AI for Disaster Preparedness
- January 7, 2020: 2020 CES Mobileye's Computer Vision (Replay)
- January 7, 2020: 2020 CES Mobileye's Global Ambitions Take Shape with New Deals in China, South Korea
- January 7, 2020: 2020 CES Unedited Ride in Mobileye's Camera-Driven Autonomous Vehicle
- January 8, 2020: 2020 CES How Intel AI Helps the Red Cross Generate Open Source Maps for Disaster Relief
- January 8, 2020: Mobileye Raises the Bar 2020 CES Roundup
- January 12, 2020: Intel and AREA15 Bring Experiential Retail to Life in Las Vegas
- January 13, 2020: In the News Mobileye's Autonomous Industry Leadership
- January 13, 2020: Intel Appoints Archana Deskus as Senior Vice President and Chief Information Officer
- January 14, 2020: In Intel's Overclocking Lab, Engineers Extend Limits of Processor Performance
- January 20, 2020: Counting Antarctic Penguins with AI
- January 21, 2020: Intel Board of Directors Elects New Chairman and New Director
- January 23, 2020: Intel Reports Fourth-Quarter and Full-Year 2019 Financial Results
- January 24, 2020: Opinion Intel's Vida Ilderem Discusses Innovations to Realize the Full Potential of 5G in Nature
- January 27, 2020: Intel, Atos and HLRN Advance Research in Earth Sciences, Fluid Dynamics and Healthcare
- January 29, 2020: In the News 'The Age of AI' Visits Kenya to See the TrailGuard AI Anti-Poaching Solution in Action
- February 3, 2020: Laika's Oscar-nominated 'Missing Link' Comes to Life with Intel Technology
- February 4, 2020: A Visit to The Sinclair, an All-Digital Tech Hotel
- February 4, 2020: Intel and Sinclair Holdings Build First All-Digital Hotel for a Greener and More Personal Experience
- February 4, 2020: Seman-Tov School Bus Fleet Lowers Collision Rates with Mobileye
- February 10, 2020: Intel Builds 10 Million QLC 3D NAND Solid-State Drives
- February 11, 2020: Up Close with Lakefield - Intel's Chip with Award-Winning Foveros 3D Tech
- February 12, 2020: Professor Amnon Shashua and Dr. Demis Hassabis Named Laureates of the International Dan David Prize for Outstanding Contributions in the Field of Artificial Intelligence
- February 18, 2020: From Sand to Silicon The Making of a Microchip
- February 18, 2020: Intel and QuTech Unveil Details of First Cryogenic Quantum Computing Control Chip, 'Horse Ridge'
- February 19, 2020: How Does Intel Make Chips A New Video Shows You.
- February 24, 2020: Intel Announces Unmatched Portfolio for 5G Network Infrastructure
- February 24, 2020: Intel Reinforces Data Center Leadership with New 2nd Gen Intel Xeon Scalable Processors
- February 24, 2020: Navin Shenoy Introduces Intel's 5G Network Portfolio
- February 25, 2020: Datto Announces Integration Solution with Intel, Focuses on Remote Manageability Based on Intel vPro Technology
- February 25, 2020: Intel World Open Path to Tokyo Kicks off in March
- February 26, 2020: Intel Highlights Latest Security Investments at RSA 2020
- February 26, 2020: Product Fact Sheet Accelerating 5G Network Infrastructure, from the Core to the Edge
- March 2, 2020: Billions of Transistors Make Up a Processor. A New Video Shows How They are Made.
- March 5, 2020: Intel Demonstrates Industry-First Co-Packaged Optics Ethernet Switch
- March 9, 2020: Tour the Intel Museum in a New Video
- March 10, 2020: Intel's Minibots Earn A Spot on Fast Company's Most Innovative Companies List
- March 12, 2020: Intel Declares Quarterly Cash Dividend
- March 13, 2020: Intel Update Managing the Coronavirus Situation
- March 16, 2020: Computers That Smell Intel's Neuromorphic Chip Can Sniff Out Hazardous Chemicals
- March 16, 2020: How a Computer Chip Can Smell without a Nose
- March 18, 2020: Intel Announces Unmatched Portfolio for 5G Network Infrastructure
- March 18, 2020: Intel Scales Neuromorphic Research System to 100 Million Neurons
- March 19, 2020: Intel CEO Letter to Customers 'We are here for you'
- March 23, 2020: Intel Donates More Than 1 Million Protective Items for Healthcare Workers in Coronavirus Fight
- March 24, 2020: DarwinAI Makes AI Applications More Efficient and Less of a 'Black Box' — with Its Own AI
- March 26, 2020: Intel Allocates $6 Million for Coronavirus Relief, Builds on Previous Efforts
- March 30, 2020: Intel Statement on New Tokyo Olympics Date
- March 31, 2020: Intel CEO Bob Swan's Annual Letter
- April 2, 2020: 10th Gen Intel Core H-series Introduces the World's Fastest Mobile Processor at 5.3 GHz
- April 7, 2020: Intel Commits $50 Million with Pandemic Response Technology Initiative to Combat Coronavirus
- April 9, 2020: Intel Joins Georgia Tech in DARPA Program to Mitigate Machine Learning Deception Attacks
- April 14, 2020: Intel Ignite Selects Next 10 Game-Changing Companies for Startup Growth Program, Moves Program Online
- April 15, 2020: Intel and QuTech Demonstrate High-Fidelity 'Hot' Qubits for Practical Quantum Systems
- April 16, 2020: Building an Intel NUC 9 Extreme Kit
- April 16, 2020: Intel and Udacity Launch New Edge AI Program to Train 1 Million Developers
- April 21, 2020: Using Artificial Intelligence to Save Coral Reefs
- April 22, 2020: Intel Offers Ultimate Intel Olympic Games Tokyo 2020 Experience
- April 22, 2020: Intel Reaches 1 Billion Gallons of Water Restored
- April 23, 2020: Intel Reports First-Quarter 2020 Financial Results
- April 24, 2020: Intel's 2020 Inventor of the Year Pushes Past Limits for Next-Gen Wireless
- April 27, 2020: Intel Provides Trusted Foundation for Azure Confidential Computing
- April 28, 2020: PC Modernization is Essential to the Future of Work, Study Finds
- April 29, 2020: Intel and MIC Announce Scale to Serve Program to Rapidly Expand Remote ICUs to 100 US Hospitals
- April 30, 2020: A New Power Standard has Desktops Sipping Energy when Idling
- April 30, 2020: More Powerful Edge Processing with 10th Generation Intel Core Processors for IoT and Edge
- May 4, 2020: There is More to Our Moovit Acquisition Than Meets the Eye
- May 5, 2020: HP Announces New Chromebooks with 10th Gen Intel Core Processors
- May 6, 2020: Microsoft Introduces New Surface Devices with Intel Core Processors
- May 7, 2020: Tile Announces Integrated Solution with Intel, Making PC Laptops Findable
- May 7, 2020: Unified Programming Model Critical to Uncompromised Application Performance, Saves Time and Money, Study Finds
- May 11, 2020: Intel Works with University of Pennsylvania in Using Privacy-Preserving AI to Identify Brain Tumors
- May 12, 2020: Intel Capital Invests $132 Million in 11 Disruptive Technology Startups
- May 12, 2020: Opinion CCS Insight Separates vRAN Fact from Fiction
- May 13, 2020: New Intel vPro Platform Enables Uncompromised Productivity and Performance for the Modern Workforce
- May 14, 2020: Closing Out a Decade of Corporate Responsibility Accomplishments and Creating Greater Impact for the Next Decade
- May 14, 2020: Intel Launches First Global Challenges, Marks a New Era of Shared Corporate Responsibility
- May 15, 2020: Technology from Intel Capital Firms Helps in the Coronavirus Fight
- May 19, 2020: Dell Technologies Announces New Business PCs with 10th Gen Intel Core vPro Processors
- May 19, 2020: 'Where Tomorrow Begins' Intel's Components Research Labs
- May 20, 2020: Intel Acquires Rivet Networks, Boosting Intel's Wi-Fi Offerings for PC Platforms
- May 21, 2020: Intel Announces 2020 US Partner of the Year Awards for Excellence in Accelerating Innovation
- May 28, 2020: COMPUTEXOnlineTalks Intel Calls for Tech Ecosystem to Come Together to Drive Innovation that Enriches Lives
- May 28, 2020: HP Introduces New Premium Commercial PCs with 10th Gen Intel Core vPro Processors
- May 28, 2020: Mobileye president and CEO Prof. Amnon Shashua explains why Mobileye is taking a redundant sensing approach to its autonomous vehicle system design.
- May 31, 2020: Bob Swan Memo The Sidelines are Not an Option; Intel Pledges $1M
- June 10, 2020: Intel Hybrid Processors PC Experiences for Form Factors Like Foldables, Dual Screens
- June 11, 2020: Changes in Intel's Technology, Systems Architecture and Client Group (Update)
- June 15, 2020: 2020 VLSI Symposia Intel Displays Intelligent Edge, Energy-Efficient Performance Research
- June 15, 2020: Intel CET Answers Call to Protect Against Common Malware Threats
- June 16, 2020: Intel Named to 100 Best Corporate Citizens of 2020
- June 16, 2020: Intel RealSense Depth Camera D455 Twice the Range, Better Performance
- June 17, 2020: Intel Elects Dion J. Weisler to Board of Directors
- June 17, 2020: Intel's Asha Keddy Discusses Future Communications Infrastructure Technologies to Meet Network Demands
- June 18, 2020: Accelerating AI and Analytics - Intel Processors, FPGAs, Memory & Storage, and Software
- June 18, 2020: Intel's AI and Analytics Platform with New Processor, Memory, Storage and FPGA Solutions
- June 19, 2020: Lakefield Hybrid CPU with Foveros Technology
- June 21, 2020: Swedish e-Science Research Center Announced as Intel's First oneAPI Center of Excellence, Focuses on Accelerating GROMACS
- June 22, 2020: Four Team USA Athletes Join Team Intel
- June 23, 2020: Sharpening Our Research Focus for the Needs of a Post-Pandemic World
- June 25, 2020: AI for Youth Uses Intel Technology to Solve Real-World Problems
- June 25, 2020: Intel Launches First Artificial Intelligence Associate Degree Program
- June 25, 2020: Intel, National Science Foundation in Wireless-Specific Machine Learning Edge Research
- June 26, 2020: Intel and SK Telecom Join Forces to Deliver on 5G's Promise
- June 30, 2020: Intel and the International Olympic Committee to Provide Support Services to Athletes
- June 30, 2020: Intel Contributes Advanced oneAPI DPC Capabilities to the SYCL 2020 Provisional Spec
- July 2, 2020: Intel Capital to Invest in Jio Platforms
- July 7, 2020: Mobileye and WILLER Partner on Self-Driving Mobility Solutions for Japan, Southeast Asia
- July 8, 2020: Introducing Thunderbolt 4 Universal Cable Connectivity for Everyone
- July 13, 2020: Travel Intel's Autonomous Superhighway
- July 15, 2020: Singapore Researchers Look to Intel Neuromorphic Computing to Enable Robots That 'Feel'
- July 16, 2020: Intel Declares Quarterly Cash Dividend
- July 16, 2020: Mobileye Starts Testing Self-Driving Vehicles in Germany
- July 20, 2020: Mobileye and Ford Announce High-Volume Agreement for ADAS in Global Vehicles
- July 21, 2020: Intel's PRTI at 100 Days In Los Angeles, Online Classes that Inspire
- July 23, 2020: Intel Reports Second-Quarter 2020 Financial Results
- July 26, 2020: As @NoHandsNZ Builds a Gaming PC, Intel Learns
- July 26, 2020: Making Gaming More Inclusive
- July 27, 2020: Fujitsu Announces Business Notebooks, Desktop PCs and Workstations with 10th Gen Intel Core vPro Processors
- July 27, 2020: Intel Makes Changes to Technology Organization
- July 27, 2020: Intel RealSense Technology Selected by RightHand Robotics for Automated Order Fulfillment
- July 29, 2020: A Critical Opportunity for US Semiconductor Competitiveness
- July 29, 2020: Intel Named One of Forbes' 'World's Most Valuable Brands' of 2020
- July 29, 2020: Intel, MIT and Georgia Tech Deliver Improved Machine-Programming Code Similarity System
- July 30, 2020: Intel and Google Cloud Enable ClimaCell's Weather Prediction Accuracy
- July 30, 2020: Intel and Lenovo Research Finds Tech is Essential to Driving Global Diversity and Inclusion
- August 3, 2020: Taller than the Statue of Liberty Big Blue’ Helps Intel Expand D1X
- August 4, 2020: For Businesses to Succeed in 2030, Gen Z Says No One Can be Left Behind
- August 5, 2020: Intel and VMware Extend Virtualization to Radio Access Network for 5G
- August 6, 2020: MediaTek Sampling Intel 5G Modem Solution for Next-Gen PC Experiences
- August 11, 2020: Dell Launches New Latitude Chromebook Enterprise with 10th Gen Intel Core Processors
- August 11, 2020: Intel Launches Expanded Initiative to Help Underserved Students, School Districts Overcome COVID-19 Barriers
- August 11, 2020: Intel Optane Persistent Memory DAOS Solution Sets New World Record
- August 13, 2020: Architecture Day 2020
- August 13, 2020: Intel Delivers Advances Across 6 Pillars of Technology, Powering Our Leadership Product Roadmap
- August 14, 2020: Intel AI-Powered Virtual Assistant Mobilized to Assist Reopening of Military Museum
- August 18, 2020: Intel Recommends Stockholders Reject TRC Capital’s Mini-Tender Offer’
- August 19, 2020: Intel and Accenture Support Neuromorphic Research Project to Assist Wheelchair-Bound Pediatric Patients
- August 19, 2020: Intel Initiates $10 Billion Accelerated Share Repurchase Agreements
- August 20, 2020: AOMedia Software Implementation Working Group to Bring AV1 to More Video Platforms
- August 20, 2020: Intel Travels to Africa to Closely Track Responsibly Sourced Tech Minerals
- August 24, 2020: Intel’s Lama Nachman and Peter Scott-Morgan Two Scientists, One a Human Cyborg’
- August 24, 2020: SIGGRAPH 2020 Intel oneAPI Rendering Toolkit Unleashes Film Fidelity
- August 25, 2020: Intel Plays Key Role in World's First Successful End-to-End Virtualized 5G Data Session
- August 25, 2020: Watch Raja Koduri’s No Transistor Left Behind’ Keynote at Hot Chips 2020
- August 26, 2020: Intel Collaborates with Argonne National Laboratory, DOE in Q-NEXT Quantum Computing Research
- August 27, 2020: Houston Depends on Intel Tech for Healthcare, Education and Connectivity Solutions
- August 27, 2020: Intel Response to COVID-19 Crisis
- August 31, 2020: Intel Studios Showcases Volumetric Production at 77th Venice International Film Festival
- September 1, 2020: Intel Promotes Chief Diversity and Inclusion Officer Barbara Whye
- September 2, 2020: Intel Launches World’s Best Processor for Thin-and-Light Laptops 11th Gen Intel Core
- September 2, 2020: Live Blog Intel Launch Event 2020
- September 2, 2020: Sparking the Next Era for the Intel Brand
- September 10, 2020: Diversity and Inclusion Read Barbara Whye’s Op-ed; Intel-Lenovo Research
- September 10, 2020: Intel Powers Samsung Medison’s Fetal Ultrasound Smart Workflow
- September 11, 2020: 11th Gen Launch
- September 11, 2020: Investing in Intel’s Manufacturing to Win in Product Leadership
- September 14, 2020: Intel Ignite Expands to Austin, Texas, and Munich, Germany
- September 15, 2020: Intel Named One of the 100 Best Companies for Working Mothers
- September 15, 2020: Intel, Baidu Drive Intelligent Infrastructure Transformation
- September 16, 2020: Intel Foundation in Million Girls Moonshot’ for STEM Diversity
- September 17, 2020: Intel’s Biggest Water Facility Hits a Milestone 1 Billion Gallons Recycled
- September 18, 2020: Intel Declares Quarterly Cash Dividend
- September 22, 2020: 10nm Intel Xeon Scalable Processors to Power Oracle's Next-Gen HPC Cloud
- September 22, 2020: Intel Appoints Saf Yeboah-Amankwah as Senior Vice President and Chief Strategy Officer
- September 23, 2020: Aternity, Intel Partner to Enhance Digital Employee Experience
- September 23, 2020: Intel Fuels the Edge Today with Expanded Tech, Customer Deployments
- September 23, 2020: Intel Industrial Summit 2020
- September 23, 2020: IoT-Enhanced Processors Increase Performance, AI, Security
- September 23, 2020: Mobileye, Geely to Offer Most Robust Driver-Assistance Features
- September 23, 2020: UAE to Get Auto Safety Tech from Israel-Based Mobileye
- September 23, 2020: Why the Geely Auto Group Win is a Game Changer
- September 27, 2020: Intel Enters into Strategic Collaboration with Lightbits Labs
- September 27, 2020: Prosthetic Hand Builds Street Cred and a Lasting Friendship
- September 30, 2020: Opinion Barbara Whye Introduces New 'Global Inclusion Index'
- October 1, 2020: Intel Expands 5G Network Infrastructure Offerings in a $25B Market
- October 2, 2020: Building a Future for Semiconductor Manufacturing in America
- October 2, 2020: Intel and Sandia National Labs Collaborate on Neuromorphic Computing
- October 2, 2020: Intel Celebrates Manufacturing Day
- October 2, 2020: Intel Wins US Government Advanced Packaging Project
- October 5, 2020: Intel Optane Persistent Memory Wins a Best of VMworld 2020 Award
- October 6, 2020: Advancing the Telehealth Revolution Through Enabling Technologies
- October 12, 2020: Building More Secure Technology with Intel SDL
- October 12, 2020: IEEE Quantum Week Intel Highlights Full-Stack Quantum Research
- October 12, 2020: Intel India, Government, Academics Launch AI Research Center
- October 12, 2020: Intel on 100 Most Sustainably Managed Companies
- October 13, 2020: Measuring Packages and Pallets at the Speed of Light
- October 14, 2020: Intel Named One of America's Most JUST Companies
- October 14, 2020: Intel SGX Helps UCSF Propel Medical Device Innovations
- October 14, 2020: Intel Xeon Scalable Platform Built for Most Sensitive Workloads
- October 14, 2020: NEC Creates a Confidential Computing System Backed by Intel SGX
- October 15, 2020: AI is Essential to India’s Future of Work, Study Finds
- October 15, 2020: Intel and Argonne National Laboratory Celebrate Exascale Day
- October 15, 2020: Smart, Programmable Interconnects Unleash Compute Performance
- October 19, 2020: Intel and Katana Graph Team on Large-scale Graph Analytics
- October 19, 2020: SK hynix to Acquire Intel NAND Memory Business
- October 20, 2020: Google Launches Parallels for Intel-Based Chromebooks
- October 20, 2020: Intel Powers First Satellite with AI on Board
- October 22, 2020: Intel Reports Third-Quarter 2020 Financial Results
- October 28, 2020: Lenovo-Intel Study The Right Technology Empowers Employees
- October 29, 2020: Intel to Acquire SigOpt to Scale AI Productivity and Performance
- October 29, 2020: Intel’s 11th Gen Processor (Rocket Lake-S) Architecture Detailed
- October 31, 2020: Innovation Extends with Intel Iris Xe MAX Graphics and Deep Link
- November 2, 2020: DISH Selects Intel for Its Groundbreaking 5G Buildout
- November 2, 2020: Intel’s Biggest Virtual Launch Tiger Lake Unveiled Amid COVID-19
- November 5, 2020: First Wave of Intel Evo Verified Designs
- November 10, 2020: Intel Promotes Tara Smith to Chief Communications Officer
- November 11, 2020: Intel Executing toward XPU Vision with oneAPI and Intel Server GPU
- November 11, 2020: Tencent Teams with Intel on XianYou Cloud Gaming Platform
- November 12, 2020: LLNL's New 'Ruby' Supercomputer Taps Intel for COVID-19 Research
- November 16, 2020: Intel’s Diverse Technologies in New Mexico
- November 17, 2020: First Intel Structured ASIC for 5G, AI, Cloud and Edge Announced
- November 17, 2020: FPGA Technology Day 2020
- November 17, 2020: Intel and Argonne Developers Carve Path Toward Exascale
- November 17, 2020: Intel Building the Future of High Performance Computing
- November 17, 2020: New Intel Open FPGA Stack Eases Development of Custom Platforms
- November 17, 2020: Supercomputing 2020
- November 18, 2020: Crypto Acceleration Enabling a Path to the Future of Computing
- November 18, 2020: Survey Shows Next Era of Healthcare Will Be Powered by AI
- November 19, 2020: Dan Rodriguez 5G to Transform Technology, Business
- November 23, 2020: Bob Swan Open Letter to President-elect Biden
- November 30, 2020: Intel and Google Cloud Simplify Hybrid and Multi-Clouds Deployment
- December 1, 2020: AWS Leverages Habana Gaudi AI Processors
- December 3, 2020: Intel Advances Progress in Integrated Photonics for Data Centers
- December 3, 2020: Intel Debuts 2nd-Gen Horse Ridge Cryogenic Quantum Control Chip
- December 3, 2020: Intel Labs Day 2020
- December 3, 2020: Intel Machine Programming Tool Detects Bugs in Code
- December 3, 2020: Update on Intel’s Neuromorphic Ecosystem Growth and Progress
- December 8, 2020: Intel and Consilient Join Forces to Fight Financial Fraud with AI
- December 8, 2020: New Intel oneAPI Toolkits for XPU Software Development
- December 14, 2020: Intel Named One of WSJ's Management Top 250
- December 15, 2020: AbbVie Uses Intel AI Tech to Improve Research Translations
- December 15, 2020: Mobileye AVs Can Go Anywhere in Germany
- December 15, 2020: We Must Collaborate, Not Compete, on Diversity
- December 16, 2020: Intel Announces Its Next Generation Memory and Storage Products
- December 16, 2020: Memory & Storage Moment 2020
- December 17, 2020: Building the Industry’s Best PC Experiences
- December 17, 2020: Intel’s 2021 Predictions for the Edge
- December 17, 2020: Project Athena An Innovation Program
- December 17, 2020: Samsung Achieves 305Gbps on Its 5G SA Core
- December 18, 2020: IEDM 2020 Marks Key Process and Packaging Innovations by Intel
- December 18, 2020: Interconnect Technologies
- December 18, 2020: Real-Time Network Visibility is Critical for Data Centers
- December 21, 2020: 2020 Intel Industry Analyst Summit Highlights
- December 23, 2020: 10nm, Creative Improvements Expand Intel Manufacturing Capacity
- December 23, 2020: Intel’s 2020 Year in Review
- December 28, 2020: CDW and Intel Partner to Simplify the Complex for Customers
- December 28, 2020: With Intel Tech, A Singapore Coffee Shop Builds a Robot Barista
- December 29, 2020: Intel Corporation Statement Regarding Third Point LLC Letter